A lesson in using mortality data to properly inform public health policy (England Mortality, 2014 to 2022)
An analytical approach to contextualising excess mortality during the COVID-19 era with respect to prior, broadly accepted fundamentals of virology, population health, govt policy, and "vaccines".
This article can be read in two parts.
The preface is a précis of an article written by Dr Howard David Stupak which I find to be a rather elegant and clear explanation of the context of COVID and framework for those who are not so familiar with the basics of the virology.
The second part analysis is based on a special set of mortality data produced by the ONS, spanning the period between June 2014 and May 2022, broken down by age and sex.
If you are familiar with the basic COVID context, you can jump straight to the second part but you might still find it a useful refresher all the same. I did!
Preface
With reference to this excellent article from June 2020 by Dr Howard David Stupak, I will expand upon his arguments using the best quality mortality data from England that is yet available1.
In reality, the illness [SARS-CoV-2 ==> COVID-19] has to be governed by the laws of physics and biology, instead of the politics, randomness, and wishful thinking that seems to prevail our discussion. The goal of this article is to provide a unified concept of how weather, overall health, populations, distancing and incidence curves fit together!
Let’s start by clarifying some terms with regards to Covid-19 that have created confusion for many, including journalists:
Exposure - contact but not necessarily disease;
Severity - once exposed, disease results in minor symptoms or hospitalization and death;
Incidence - new cases per day or viral generation;
Prevalence - cumulative cases in a region or group once a measurable time period is complete.
By confusing these terms, or even using them interchangeably, headlines can create unnecessary fear or complacency where the reader reads something, but imagines another.
For example, the “grim milestone” headlines, that are usually discussing world or national prevalence crossing a numeric threshold, and are simply cumulative cases, can cause a reader to panic un-necessarily.
In other cases, a rising incidence (daily new cases) makes others concerned that the virus has entered “exponential growth” and will continue to increase unchecked until lockdowns can stop growth.
In reality, based in part on the work of a pre-germ theory scientist, William Farr, we know that pandemics and epidemics follow a roughly Bell-shaped curve in incidence. In other words, the incidence accelerates over time or host generation before peaking and then decelerating until it has decayed to an incidence of nearly zero!
However, the number of people that are susceptible may not be variable, and may remain fixed. This was evident in many of the cases where there was a coronavirus outbreak on isolated ocean-going ships, and there was a very specific amount of those who tested positive, a much smaller percentage of those who developed severe disease needing hospitalization, and an even smaller percentage of those who died.
In other words, not all of the population is even susceptible to disease, and those who are, may be only susceptible to a specific severity of disease (i.e. no symptoms, severe, or death).
Remember the days when Chris Whitty used to tell the truth? He said pretty much the same thing during the Downing Street Press Conference on 11th May 2020:
A significant proportion of people will not get this virus at all, at any point in the epidemic…
Of those who do, some of them will the get the virus without even knowing it…
Of those who get symptoms, the great majority - probably 80%, will have a mild or moderate disease. Might be bad enough for them to go to bed for a few days, not bad enough to have to go to the doctor.
An unfortunate minority will have to go to hospital but the majority will just need oxygen and then will leave hospital. And then, a minority of those will end up having to go to severe end critical care and some of those, sadly will die…
And even in the very highest risk group, the great majority, if they catch this virus, will not die.
This bit starts at 46.02 but it’s well worth listening to the whole 57 minutes of other nonsense muttered by Johnson and Vallance, and Whitty himself based on the mistaken (?) belief that transmission of an airborne virus (and therefore the COVID epidemic) can be controlled.
Also, bear in mind what I’m going to show below is that this was evident from the data at the time of their broadcast, even without the benefit of hindsight.
To the detriment of society, when people like Dr Stupak and me highlighted the manifest errors made by alleged Experts™ like Whitty and Vallance, as early as June 2020, we were ridiculed, vilified and eventually just censored2. P.S. I have it on record that I made both my constituent MPs aware of these manifest errors and their repercussions throughout. Caveat Julie Marson and Kemi Badenoch!
Thus, prevalence, or the total number of people that are susceptible to a given severity may be fixed in advance! So, only incidence, or how many new cases occur on a specific day, or in a host generation is variable per a specific population.
The “flatten the curve” campaign illustrates this well; that epidemiologists knew the final prevalence or total number susceptible and eventually affected would remain fixed and inevitable.
What does vary between communities like cities or even neighbourhood are two factors:
First, the proportion of a set of individuals who are susceptible to specific severity outcomes depends on the long-term state of the health of the respiratory system and its associated “lining” or mucosa of that individual;
Second, is the “reproduction number” (R0) for that given susceptible population for a pathogen, which depends on how efficiently a virus can be transmitted from person to person taking into account viral characteristics, concentration (crowding or distancing) of the population, as well as how many are susceptible in the population in question.
In other words, the final outcome of the impact of COVID is pre-determined. The extent to which this outcome can be delayed is the experiment undertaken in lockstep by most countries around the world through the introduction of a wide range of non-pharmaceutical interventions which were predicted to be ineffective, even by one of the most corrupt organisations associated with the COVID epidemic, the WHO.
As we will see, the experiment failed. Completely. This should come as no surprise, given that contact tracing, quarantine of exposed individuals, entry and exit screening, and border closures were “not recommended in any circumstances” in terms of epidemic or pandemic severity, let alone quarantine of healthy individuals!
One benefit of this experiment is that it has allowed us to prove that all of the other authoritarian, non-evidenced based measures are ineffective too (see Table 1 below).
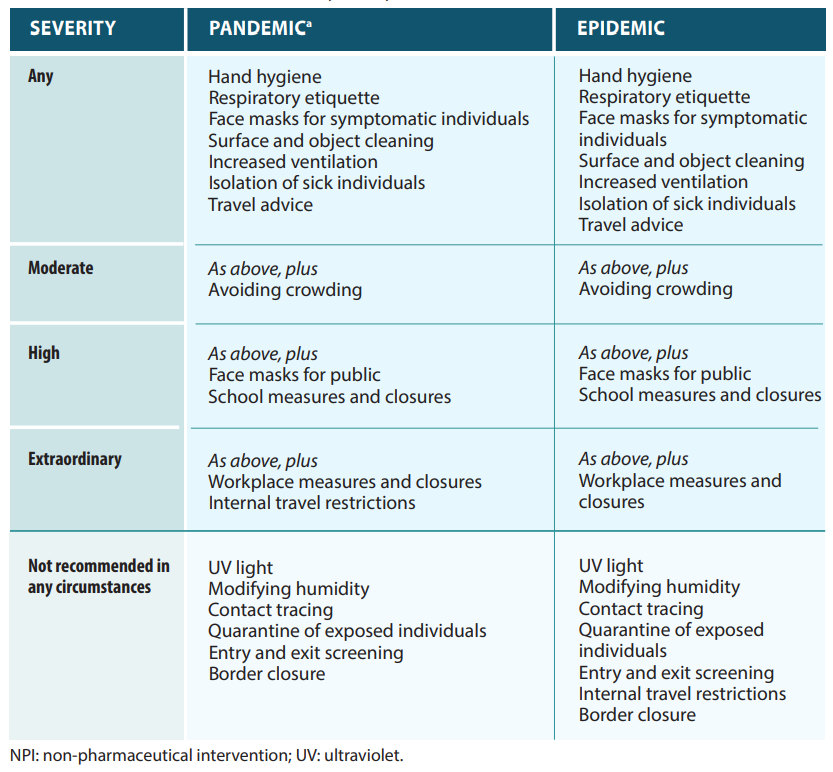
As Dr Stupak further explains:
The second variable in the “fixed prevalence” model is the efficiency of exposure. Again, assuming that the virus, like most respiratory pathogens is ubiquitous or omnipresent, the number of people eventually exposed is not variable, but only the efficiency by which with they will be exposed.
This characteristic is represented as the “reproduction number” (R0) for that given susceptible population (determined above) for a pathogen, which depends on how efficiently a virus can be transmitted from person to person taking into account viral characteristics as well as concentration (crowding or distancing) of the population in question.
So, measures like social distancing, mask-wearing, or having a very spread-out or concentrated population will effect this viral reproduction efficiency (R0), and exposure can be rapid, or very slow, but will not affect the eventual number of total cases, assuming no vaccine intervention is available.
On this last point, based on extensive analysis of incidence and severe disease, I disagree with Dr Stupak, the WHO and the assumptions of disaster modeller-in-chief, Professor Ferguson3. My comprehensive debunking of the latter's assumptions in July 2020 has stood the test of time, despite the lack of availability of good quality data at the time and my own relatively inferior analytical capabilities4. There is no evidence that any social distancing measures have had a benefit. I have argued in the past that this is most likely due to the fact that virus circulating in the population is inconsequential when most of the damage is done nosocomially!
So then, why does the disease incidence (daily cases) over time seem to first go up nearly exponentially, then peak, then decline exponentially? Because of the interplay of the two factors we just discussed (transmission efficiency and fixed prevalence of severity populations). This can be demonstrated mathematically, using Farr’s Law, describing incidence decay in a vulnerable population.
Here, I'm going to digress from Dr Stupak’s explanation which describes the situation in terms of a Bell Curve because I believe that the Gompertz Curve is the more accurate representation5. And also, furthermore, because I don't think transmission efficiency is the material factor. In fact, I think the Gompertz function captures little more than the varied susceptibility of the population.
However, Dr Stupak’s insights remain valid and consistent:
This is why northern, crowded cities (New York City for example) with cold, dry winter climates peak early and rapidly, and then have a precipitous decline, due to both incidence day as prevalence is approached and the season changes to a more favorable one to mucosal health, decreasing the proportion of susceptible individuals for the subsequent season.
In contrast, less dense cities like Phoenix will have a decreased R0 or transmission efficiency, and has a lowest humidity period in the summer. Thus, Phoenix will experience a flatter more prolonged curve with lower daily incidences, and can expect maximal prevalence in its hot, dry summer.
Hope-Simpson6 anyone?
His career-long interest in the manner of transmission of the influenza virus was first stirred by the great epidemic of 1932–33, the year in which he entered general practice. It culminated in a book, published in 1992, which questioned the theory of person-to-person transmission being enough to explain the simultaneous appearance of influenza in places far apart. His initial hypothesis proposed that the cause of influenza epidemics during winter may be connected to a seasonal influence.
Nothing “novel” here.
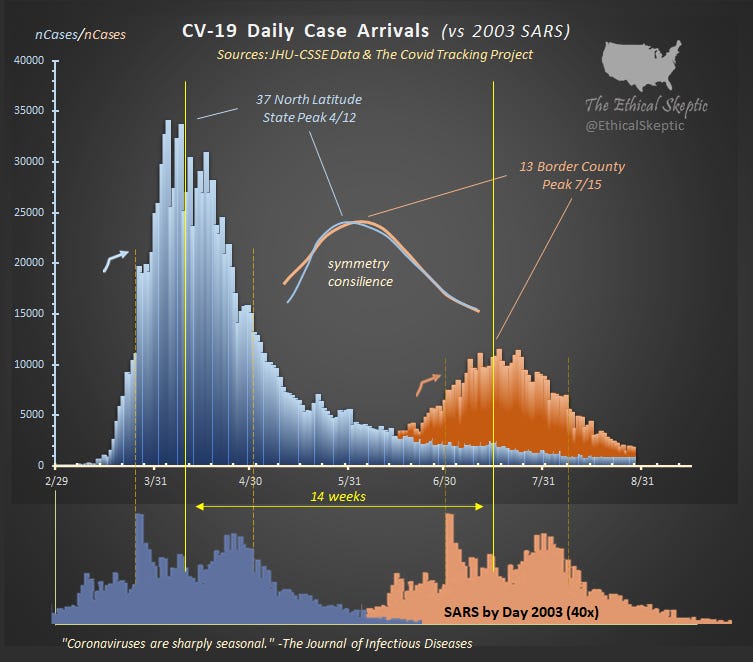
Back to Dr Stupak’s hypothesis:
In other words, as the epidemiologists have stated all along, distancing rules will not affect eventual prevalence of disease, which remains fixed, but it will lower the R0 or efficiency of transmission, prolonging the duration, but lowering the daily incidence.
Alas, as I said, and will demonstrate (soon!), this is simply not the case for much of the northern hemisphere, certainly in the west, and to a certain extent to the east. Perhaps, it has some truth for Oceania, which is a special case, but they are “getting theirs” now, as expected.
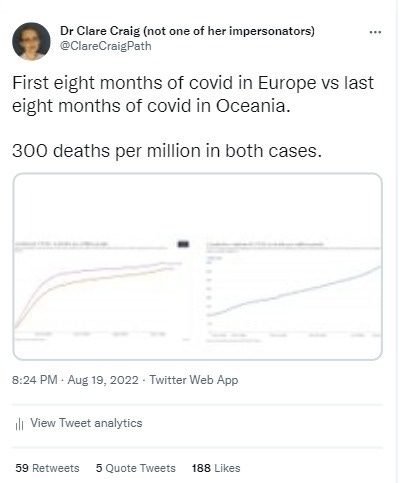
So, assuming we cannot control the eventual fixed prevalence per season, and that our society has already figured out how to balance distancing with productivity to maintain an R0 that will prevent overwhelming of hospitals and morgues, what strategies can we use to protect our cities using basic, common sense in future seasons?
A “second wave” can consist of either an incomplete prevalence curve that was initially stunted by distancing, but was eventually going to run its course, or can represent a newly susceptible group as a region enters a new climate season that worsens mucosal or airway health, if it was not exposed in the prior one.
You can read Dr Stupak’s complete Medium article for the rest but I’m grateful for his work which saves me the “writing” bit of this analysis that those of you who know me, will already know I don’t much enjoy!
Before finally getting to my analysis, a reminder that this preface was written well over two years ago. There is no defence against the enormous societal damage caused by the UK government’s and its advisers’ experimental policies, since we proved that they were ineffective before the summer of 2020, and the full, worst extent of COVID was experienced before any alleged benefit of the equally experimental “vaccine” was introduced. Note bene Rishi Sunak et al!
Analysis
This is going to get quite technical as we dive deep into forensic analysis of the England mortality data between June 2014 and May 2022, stratified by age and sex.
Consequently, I’m only going to share analysis of two age groups, otherwise this article is going to get too long to digest (it might already be as I reread it!). However, I might write a comprehensive paper and publish it on preprint server - Researchgate - if I can find the time. I doubt any of the peer-reviewed journals would touch it.
Ages 75+
The seasonal pattern of mortality for the over 75s in England is evident (Figure 1), with more deaths occurring between Sept and May each year, distributed in accordance with the Gompertz function. The summer baseline (June to Aug) has been rising slightly over the observation period.
The epidemic COVID era (spring 2020) is also very much apparent. Although it is impossible to determine the extent to which the virus or the response to the virus is responsible for these unexpected deaths, it is clear that the mortality event is over by the end of the usual mortality season. As such, I mark this period simply as “COVID”.
The endemic COVID era (starting autumn 2020) is not characterised by anything particularly unusual for the expected seasonal mortality pattern until winter 2021, coinciding with the experimental mRNA mass injecting campaign. Consequently, I label this period “mRNA” because, as you will see from the rest of this analysis, I am confident that there is more than sufficient evidence to attribute causality to the experiment.
In the “post-COVID” (in terms of the actual virus at any rate, if not the policy response) era, summer 2021 onwards, deaths in the over 75s, the group almost singularly affected by the virus, appear to have returned to pretty much normal levels and pattern.
Looking at the cumulative, excess seasonal mortality for the over 75s in England (Figure 2), we can observe that 2019/20, the COVID epidemic year, was middling in terms of mortality until 13th March 2020. By the end of the period two months later, 49,113 excess deaths over the expectation set by the forecast summer baseline have occurred. In the absence of this event, it appears that 2019/20 would have ended the period around 41,000 seasonal deaths, so it is a good argument, in fact, that 43k is the unexpected tally associated with the epidemic COVID era.
The start of the next mortality season (2020/21) is characterised by an unusually steep (albeit usually steady) rise in deaths between 22nd Oct and 31st Dec. By the end of this particular period, there have been 28,801 seasonal deaths, 14,407 more than usual. Again, these unexpected deaths have typically been attributed to COVID. However, in my opinion, they do not share the characteristic pattern of a viral outbreak but rather the more systematic rise you would associate with a more general condition of increased mortality. As such, I would argue that these are the first signs of the collateral deaths resulting from the COVID interventions earlier in the year, as much as residual COVID deaths.
The characteristic pattern of event-triggered mortality does occur in this season but much later than usual, at the start of winter 2021. This is in spite of so much excess death at the tail end of the 2019/20 season and higher than usual mortality in the early part of this season (“pull-forward”). As Dr Stupak says, the size of the susceptible population is fixed (unless something causes the susceptibility to change of course!). In the final section of this age group analysis, I will show the likely causality of the mRNA campaign for this unexpected rise in deaths.
Nevertheless, the final tally for 2020/21 is still significantly lower than 2019/20, most likely due to the “pull forward” effect alluded to above.
Finally, in 2021/22 and 2022/23, deaths appear to have normalised in terms of levels and also pattern, apart from an obvious inflection on 1st April 2022. Using Gompertz function analysis, we will examine this point for cause as well as trying to determine the benefits or harms of COVID interventions across the whole three periods.
According to analysis using Gompertz functions to isolate the idiosyncratic distributions of death, it is apparent that an unusual mortal event manifests in the data starting on 6th March 2020 (Figure 3). In spite of the title and RHS axis label, there is no “vaccine” data because the mRNA experiment had not started during this period.
It is widely accepted that SARS-CoV-2 was circulating in England at least as early as Jan 2020 so it is reasonable to attribute this mortality distribution to COVID for infections likely to have occurred between Jan and Feb 2020, such that the COVID virus was the dominant circulating pathogen. As such, I label this distribution, coloured green in the figure, “Pre-lockdown COVID”. England did not lockdown until 23rd March. Inevitably other interventions might have affected events before lockdown (school and business closures, and other disruptions to social activity) but lockdown is considered a defining act in experimental pandemic response so it deserves recognition as the pre/post era for this analysis.
The pre-lockdown distribution naturally runs to 22nd April 2020 with no apparent reduction in the empirical data compared to the Gompertz prediction. This indicates that no interventions had a beneficial impact between Feb 2020 and April 2020. It accounts for 4,407 deaths.
Moreover, rather than seeing a reduction in the existing COVID-related mortality distribution, we actually see the emergence of a second distribution running from 23rd Mar to 12th April (coloured blue and labelled “Lockdown #1”). Curiously, this distribution occurs at the exact juncture of the most unprecedented, draconian curtailment of civil liberties and denial of availability of healthcare services ever experienced in this country. It accounts for 2,963 deaths. Already, I would argue that these deaths are just as likely, if not more likely, to be due to the policy measures rather than the virus itself.
Contrary to my argument, one might argue that the Lockdown #1 deaths were also due to the virus, resulting from infections up to early March (given the observed 3 to 4 weeks manifestation period between infection and death). However, there are a subsequent four additional distributions that start after lockdown which would lower the likelihood of that being the explanation. At any rate, assuming that those distributions are due to the virus, it certainly disproves the notion that locking down otherwise healthy people prevents new outbreaks? And if they are all the result of transmissions that started to occur prior to the interventions, the fact that none of the empirical distributions deviate from the model, is the final argument on the matter - lockdowns don’t work!
In summary, the four subsequent distributions account for 37,548 seasonal deaths, with the last distribution not starting until 27th April 2020, more than 1 month after lockdown starts. I have labelled them Lockdown COVID #1 through 3 but I have also included a distribution (coloured orange) called “COVID (Care Homes)”, since this distribution coincides with the mass exodus of sick hospital patients to secondary care facilities, together with some rather dubious treatment protocols for those residents.
I intended to have a post-lockdown mortality distribution.
But there wasn’t one.
In what should be the endemic phase of COVID, during autumn 2020, we do indeed witness longer, flatter mortality distributions as we would expect, relative to shorter, sharper ones for an epidemic (Figure 4). Perhaps, I was wrong to assume earlier that the higher than usual mortality for this season was due mainly to the spring interventions? It seems more and more likely to be a combination of both policy and virus.
There are three distributions of more epidemic quality between mid-Dec 2020 and end of Feb 2021. These follow the experimental mRNA injection campaign. Although, the first distribution is the largest and follows the smallest round of injections, this might be explained by two hypotheses:
The most vulnerable were injected first so the relative injection fatality rate may be much higher than later periods;
Healthcare workers were prioritised for injections during the early period (first few weeks of Dec 2020) which may have caused a “super-spreader” situation across the facilities where the most vulnerable were contained.
Just a couple of weeks after the first round of injections finishes, so does the excess mortality (a key Bradford Hill criteria for causality in my opinion, that has never been focused on7).
The timing of the injection campaign and concomitant rise and fall of excess deaths may all be coincidental of course, given that it is done during the high mortality season. If this argument is true, one must still question the sense of doing such an experiment at that exact time? There are so few places for policy advocates to hide I’m afraid.
Moreover, it is also abundantly clear that the second round of injections, those alleged to be Effective™ at saving lives, do not occur until AFTER all the excess deaths have already entirely subsided, let alone after the spurious 2-week window of effectiveness. Attempts by the ONS and other pro-narrative organisations and agents to use all the mortality data from Jan to May 2021 to demonstrate “vaccine efficacy” (VE) are bogus and always have been8.
In the third and final season, there is nothing much more to add (Figure 3). As I said, COVID is over by this time, even though ridiculous, ineffective policies (mask mandates and “vaccine” obligations) still circulate around the world. Nevertheless, there remains the curious coincidence between injection round 3 and the start of seasonal excess death. Again, impossible to declare definitively if this is causality or just plain bad timing!
But what of April 2022 I said I would investigate? Well, the distribution is there, starting mid-March. The booster campaign appears to be well over by then so I’m afraid, with the data that is available to me, I can’t provide an insight on this. Perhaps somebody in public health office can provide the answer? Was there a second round of boosters (as there was in Israel) and they haven’t reported the data?
So, that’s the COVID story for the elderly. Next, how has this disease of the old and frail affected the younger age groups?
Ages 45 to 49
Firstly, there is no particular reason why I chose this exact age group for the second part of my analysis except for the fact that they should be young enough not to be seriously affected by COVID but old enough that there should be enough deaths to do some meaningful analyses. As it happens, at the time of writing, I haven’t looked at any other age group, so there may well be better insights to come when I do!
Starting again with a high level observation of all deaths between June 2014 and May 2022 for this age group, we can see that the summer baseline is to all intents and purposes flat and although there is seasonality to the mortality, it is not as pronounced as the over 75s (Figure 6).
COVID (spring 2020 epidemic COVID) is nowhere near as impactful, as we would expect. In fact, 2015 and 2018 are not too far off for comparison in the last few years. First thing that strikes me though is the deaths during the mRNA era. Doesn’t look like a Safe and Effective™ intervention for this age group so far, does it?
Note also, the serious lag in data reporting. I have covered this in the methodology section below and will also address it during this analysis in a moment.
Turning our attention to cumulative, seasonal excess mortality, we can once again see the timing and impact of “COVID” (Figure 7). Curiously, once again, deaths in the 45 to 49 year olds are trending BELOW expectations right up to 19th March 2020 (red line in Figure 7). How can this be, knowing that this deadly virus (the worst one since the famous Spanish one according to Boris Johnson9) was circulating for a long time before that date?
We have already observed a late season mortality distribution in the over 75s starting on 6th March. Did it take 13 days for the same event to manifest in the younger age group? If so, how come the impact on the younger age group is so much more severe? No, it is not the virus that triggered this sudden increase in deaths, it is the trigger that occurred on 19th March - the widespread disruption to social, healthcare and welfare infrastructures.
This disruption resulted in the untimely deaths of over 400 45 to 69 year olds (assuming that the expected excess seasonal deaths tally was likely to have been closer to 200 - by looking at the chart - rather than the 659 that ultimately occurred). COVID most likely did not and would not have killed them, denial of healthcare and other policy factors more probably did.
In the second season, again we observe a similar pattern to the over 75s. There is no evidence of excess seasonal mortality right up until 24th Dec 2020. Prior to that, deaths are running at the top end of the historical range (not surprisingly given the expected after-effects of the COVID interventions) but there is no pattern indicative of a deadly pathogen.
Right up through to the end of March 2021, there is an explosion of excess mortality which then levels off through to the end of the season, indicating that these deaths were not simply pulled forward by a few weeks or even a couple of months but may not have occurred for some much longer time, well over 300 of them (659 minus 299, the seasonal average).
Moving to season 3, it is difficult to argue that “COVID” is over for this age group because the data simply isn’t there. It seems apparent that the reporting lag starts around 14th Nov 2021, perhaps earlier. At the time of writing, that indicates a backlog of eight months and possibly at least 1,100 deaths currently sitting with the coroner. I asked the coroner to confirm some time ago but have still not yet had a response10. Understandably, he is very busy.
There is however a signal of sorts between 6th Sept and 21st Oct where it looks like 40 or more deaths have been pulled forward by a couple of weeks or so? Again, I will try and investigate this later in the analysis.
Moving on now to Gompertz analysis… Figure 8 reveals that there are just two idiosyncratic death distributions using this empirical model.
As observed before, the first starts around 19th March 2020, runs through to 10th May 2020, and accounts for 378 deaths, completely unaffected by any external factor.
The second starts around 11th April 2020, also runs through to around mid-May, and accounts for 180 deaths, also unaffected by any external factors. If I was being particularly diligent, I might argue that the latter distribution is the one that correlates with “Pre-lockdown COVID” deaths of the over 75s because of the timing (one month after the older age group), shape and magnitude of the distribution but I don’t think it makes much material difference to the arguments - COVID is not a significant event for 45 to 49 year olds.
And frankly, that’s it. What I said back in July 2020 has stood the most rigorous test of time, COVID was over in England by the end of May 2020. The epidemic ran its natural course and the virus returned in endemic quality the follow autumn. If no attention was paid to it, that would have been the full “unprecedented” extent of its mortal impact.
Alas, that was not the case…
Try as they might, pro-narrative pawns like Tom Whipple and the insidious Twitter Mutton Crew™, calling people like me and Clare Craig, crazy conspiracy theorists for daring to suggest any possible link between the mass mRNA experiment and apparent increases in COVID mortality should at least be investigated, they couldn’t change the actual data. Denying the thing could possibly have happened did not stop the thing actually happening. Who knew?
In Figure 9, I have attempted to model the only somewhat meaningful excess deaths distribution prior to the experiment that we might attribute to endemic COVID but even here, I’m really clutching at straws. Weird then, that suddenly, just a couple of weeks after the mRNA experiment starts, we should experience not one but four idiosyncratic excess deaths distributions, amounting to 610 excess deaths compared to the 56 before the campaign? And that’s 60% more than this age group suffered during the epidemic, before the Safe and Effective™ even became available! 🤔
Once again, any feeble attempts to measure effectiveness are worthless given the excess deaths have already stopped, concomitantly with the ending of the experiment, many weeks before the “cure” could take effect.
Has there been any impact of the booster campaign? Again, according to Figure 10, impossible to say because the data simply isn’t yet available.
And what of the signal we detected in Figure 7 (and circled black in Figure 10)? At first glance, it looks like it is too early to be caused by the booster campaign. But is it?
On closer inspection (Figure 11), it is evident that over 70,000 boosters were given between 6th and 23rd Sept 2021, which is when the bulk of these unexpected deaths occurred. Given the apparent short duration of the pull-forward effect, it seems perfectly feasible to me that these could well be the most sick and vulnerable “prioritised” for injection.
Sex
If we break the data down by sex, can we glean any additional insight?
Looking at the first charts used in my analysis (Figure 12), we can observe that less women (biological ones) in the 45 to 49 year old age group die than men - on average 14 per day during the summer months compared to 22. Perhaps more insightful for this analysis is that it appears that the reporting lag is much greater for the men than the women. In other words, the event that is killing this age group in the last eight months is affecting men much more than women. The same could not be said for natural COVID.
Assuming 2021/22 should be an average year (conservative), the data in Figure 13, would corroborate this with 780 unreported male deaths (35 summer days worth) and 376 unreported female deaths (27 summer days worth).
Note also, in the female data, there are LESS deaths than one would expect between 1st Nov and 24th Dec 2020 (green dotted circle in Figure 13). This is during a period when we were led to believe (viz. “grim milestone” headlines based on cases), was still very much in the midst of a deadly pandemic. Excess female deaths in this age group only increase two weeks after the mass mRNA experiment (“inevitably” is going to be overused). Where was deadly COVID before then? Both sexes were equally affected during spring 2020 with deaths starting on the exact same date - 19th March 2020 - so it’s not something demographic related.
Conversely, look at male deaths during the same period, a steady climb, consistent with this time of year in prior periods but at the very top of the range. Is this indicating the first results of earlier COVID interventions, disproportionately affecting men for whatever reason? A more detailed analysis of cause of death for these men would be very helpful.
Conclusion
Using a wide variety of robust, analytical techniques and models, it is apparent that deaths of the elderly and middle-aged in England are significantly worse in the COVID mRNA era relative to the natural virus era. The evidence to suggest that this is because of the mRNA experiment, not in spite of it, is compelling.
Furthermore, the analysis also suggests that the interventions imposed upon the English citizens during the natural epidemic - the widespread disruption to social, healthcare and welfare infrastructures - may well have substantially contributed to the death toll as well, if not substantially caused it.
Finally, the impact on mortality as an adverse outcome of the COVID intervention policies may already be apparent as early as autumn 2020, especially in younger men.
This analysis could have been improved and performed more readily had vital information on deaths occurrences been made available in a more timely manner.
Both the absence of measures to improve upon the quality of the process for gathering and distributing mortality data, and the abject failure of the public health authorities or other official statistics authorities to perform honest and meaningful analyses on the the data that was available, has also most likely contributed to the overall burden of loss being much higher than it might have been if none of the COVID policies, including the mass mRNA injection campaign, had been undertaken.
Methodology
Sure enough, I had to shell out an additional £540 to get what should be routinely made available from the ONS but thankfully, my paid subscribers have already more than covered the cost.
Jessica Rose - your expert opinion, please!
From almost the beginning I said that the SARS-CoV-2 strategy was flawed, not because it was counter-intuitive in the face of previous pandemic experience and recommendations, but because it was wrongly directed. What was important was not to stop the virus spreading (because, as you have pointed out, most people don't get very sick) but to correctly treat those who do get sick. I outlined the correct treatment modalities in May 2020; I was not listened to, and a completely unnecessary trial (the RECOVERY trial) was done which proved what was already known. As time has passed the accuracy of outside predictions has become very clear and I fail to understand why so many experts who had the right credentials (I include myself) found their advice disappearing into a black hole. Covid-19 is a clinical entity, so whyits confrontation should be led by statisticians and other people not involved in clinical medicine remains a mystery to me.
"In reality, the illness [SARS-CoV-2 ==> COVID-19] has to be governed by the laws of physics and biology, instead of the politics, randomness, and wishful thinking that seems to prevail our discussion. " - a perfect summary of the whole situation.